AI In Agriculture – A ‘Smart Farm’ Introduction
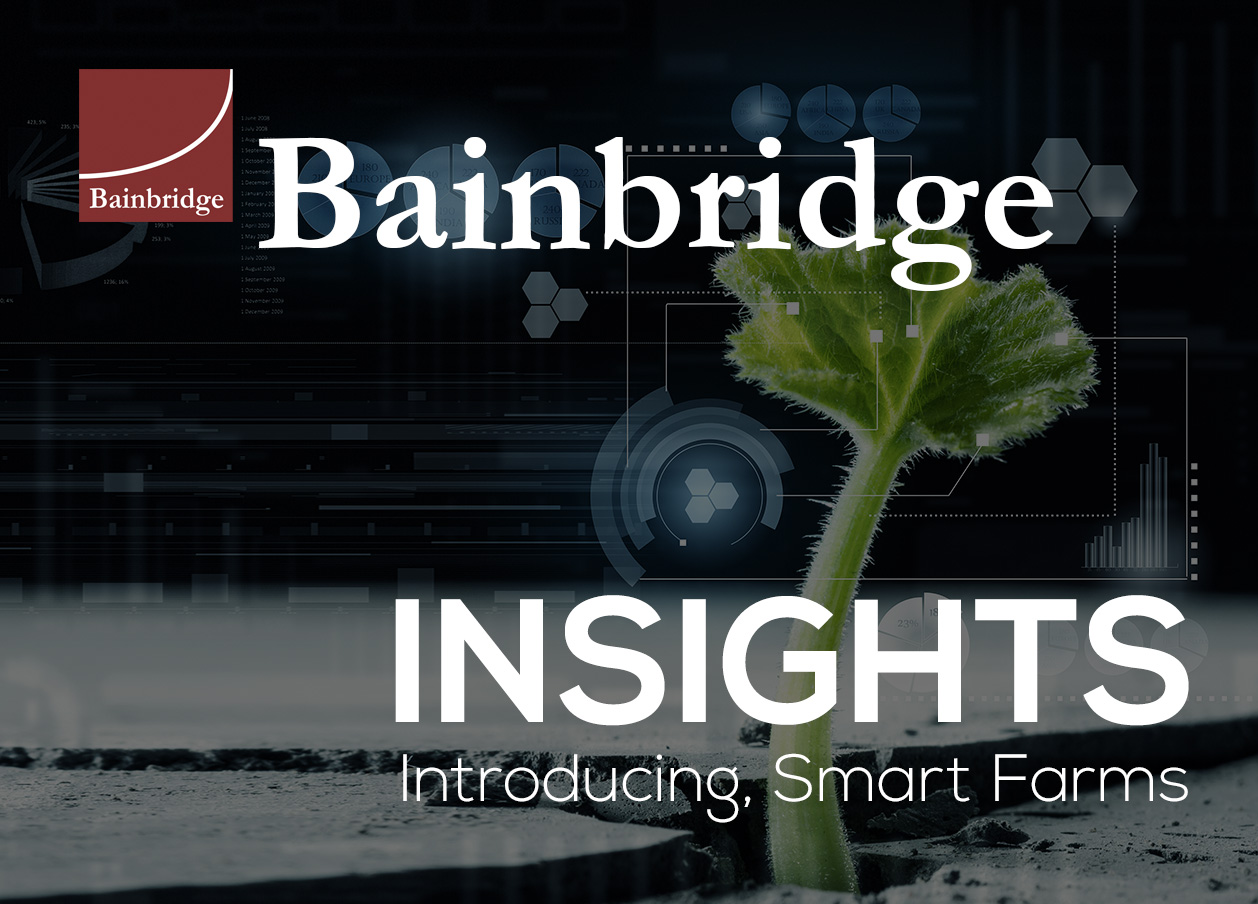
AN NEW WAY TO FARM
The potential for artificial intelligence in agriculture has been growing exponentially as the technology for this industry continuously advances. Food cultivation began adjacent to the evolution of man and remains essential to human life despite drastic shifts in the economy, society, and environment. Today, the agriculture industry stands armed with drones and robots programmed to gather data from production fields in order to advance precision agriculture or ‘smart farms’.
AUTOMATED FARMING – THE POTENTIAL
Operating as a massive five trillion dollar industry, agriculture represents 10 percent of global consumer spending, 40 percent of employment, and 30 percent of greenhouse gas emissions. Currently, machine learning in agriculture is focused on monitoring and forecasting farm productivity. Device sensors record key information about crops or livestock in real time to cross-reference the new information with pre-defined data sets that are programmed into the machine. Some devices are designed for roaming to terminate pests, plant diseases, and weeds with much more precision than humans and less environmental impact.
Interconnected technologies compute high-performance analytics to help farmers understand real time data such as weather and temperature. Farmers are even provided with insights on how to increase crop yield, improve farm planning, make environmentally intelligent decisions on the level of resources needed and where to distribute them. Farm productivity is set to improve over the next five years as cognitive computing in agriculture continues to grow bigger and ordinary farms transform into digitally controlled ‘smart’ farms. By 2020, it is estimated that over 75 million agricultural IoT devices will be in use thus opening up more opportunities for agricultural businesses. AI in agriculture will be a significant disruptor in the green technology revolution.
APPLIED EXAMPLES
What was once a theoretical concept is now a practical application for the agriculture industry. Automated irrigation systems, crop health monitoring and face recognition systems for domestic cattle are proven examples of how artificial intelligence has made a significant impact for improving farm productivity.
CROP HEALTH MONITORING
This remote sensing technique is essential to land cover performance and gathering information on farmland resource conditions. Crop health monitoring falls under the definition of agriscience which combines hyper spectral imaging and 3D laser scanning to produce enhanced information and plant metrics across thousands of acres. The spatial resolution of the technology is able to evaluate individual plots or plants and the temporal advantage of tracking the changes in the crop throughout the growing cycle as well. When comparing the traditional time consuming methods of land surveying and advanced digital detection technologies for land surveying, experts believe farmers will quickly adapt to the new technology in order to improve quality and efficiency of their crop.
AUTONOMOUS WARNING SYSTEM FOR FRUIT FLY OUTBREAKS
Massive fruit fly outbreaks result in the famer’s dependence on chemical pesticides, ultimately devaluing the farm-to-fork supply chain. An autonomous early warning system was developed to reduce the probability of these outbreaks occurring. The system was designed on a foundation of wireless GSM networks that capture long-term and real-time natural environmental fluctuations in fruit farms. Self-organizing maps and support vector machines are incorporated into the warning system to perform adaptive learning. When the system detects a potential outbreak, a warning message is sent to farmers and government officials via GSM networks.
FACIAL RECOGNITION SYSTEM FOR CATTLE
For years image classification and facial recognition programs have been incorporated into digital applications like social media. By applying advanced metrics such as body condition score and feeding patterns, dairy farms can individually monitor all behavioral aspects in a group of cows. Facial recognition can make it possible to diagnose lame cow before she shows severe symptoms thus saving the farmer months of low milk production. The system would measure the cow’s back to detect early signs of the lameness for the farmer. Data within the system can also determine what type of feed a certain cow can eat. This allows farmers to optmizie their inventory and decrease cost on feed.
AI IN AGRICULTURE – WHAT’S NEXT?
There are a few limitations on the widespread deployment of artificially intelligent robots in farm fields including power and the strain of outdoor weather conditions. The devices will need a charging platform and battery life strong enough to survive hours of operating in the field. In addition, materials will need to be constructed that can cope with the unpredictable chance of rain, wind, and dust on farm fields. There are issues within programs that can identify decisions in the field but can’t analyze them in real time like a human can. More so, these programs are unable to autonomously put decisions into practice or create predictive analytics – the true nature of machine learning in artificial intelligence.
However, the next step is to combine more complex machines, improve contextual data collection techniques and highly developed software. Experts believe these improvements are not too far away from coming into fruition for large-scale implementation. Farmers are eager for this technology to relieve some of the inevitable challenges in agriculture.